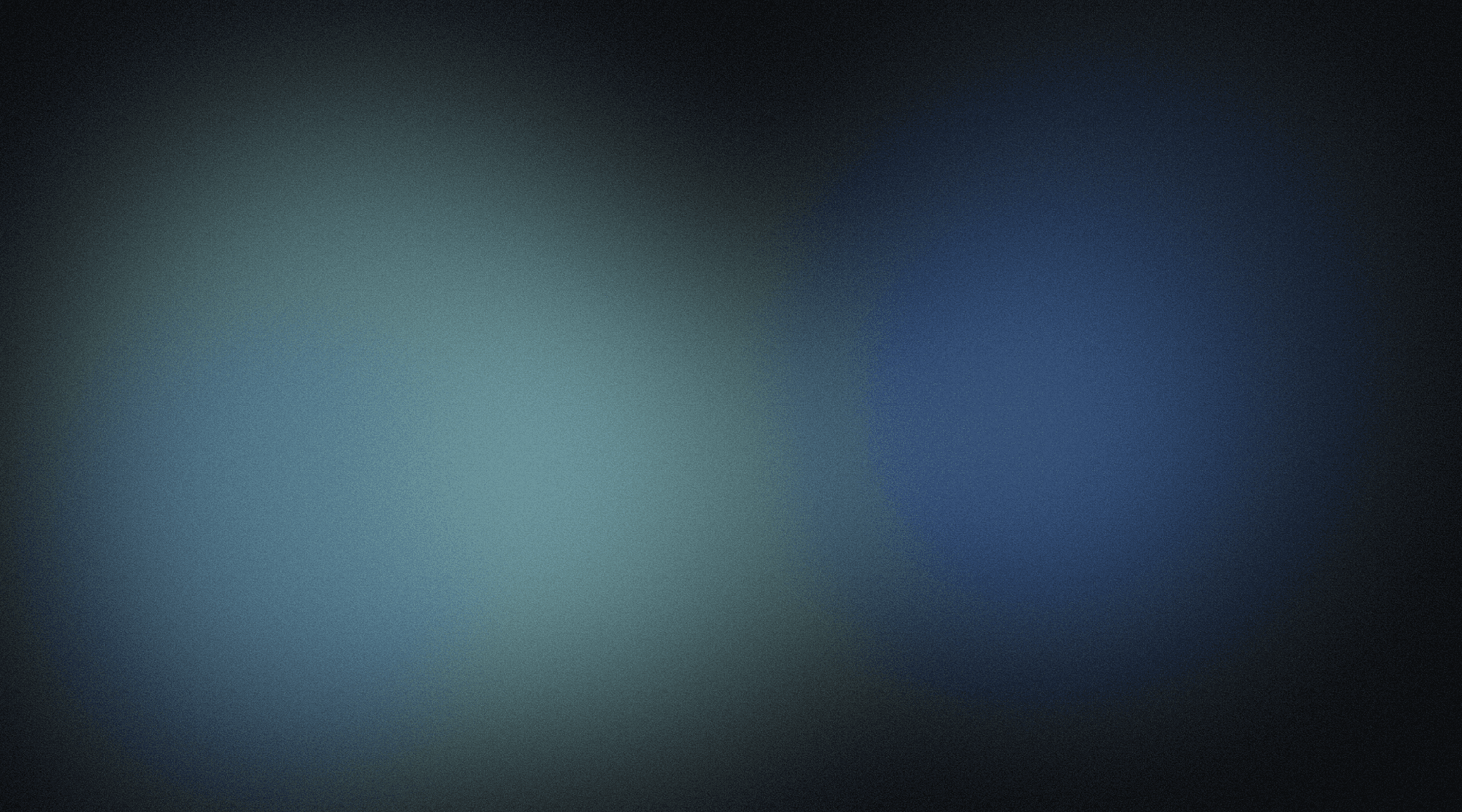
In today's competitive business landscape, efficient customer communication is key. However, many platforms lack features for effective team collaboration, leading to delays and reduced customer satisfaction.
Front addresses these issues with an innovative platform that integrates multiple communication channels and automates workflows. This improves response times and team efficiency, thereby enhancing customer satisfaction and retention — key drivers of revenue growth.
Building on this, I've decided to explore the potential of further automation to enhance the speed and efficiency of our customer support teams.
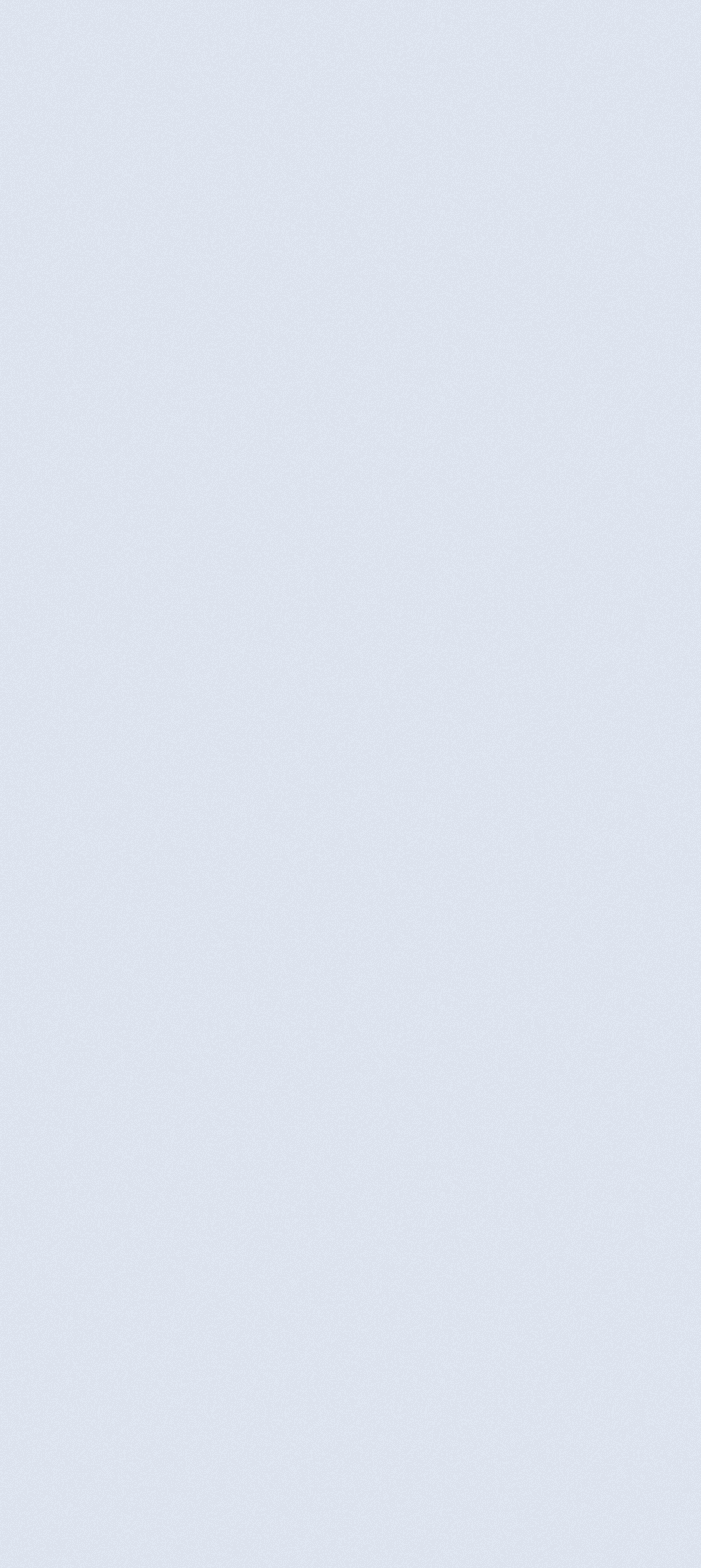
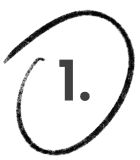
Power users of customer service tools like Intercom, Zendesk, and Front are seasoned customer support professionals who manage a high volume of interactions across various platforms.
They want to be effective and respond quickly and correctly to keep customer satisfaction and retention. All tools are trying to help them being more efficient and optimize customer experiences while managing workflows efficiently.
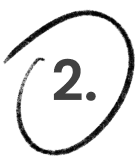
better understand how we can enhance their workflow, it's important to first analyze their current processes. This will allow us to identify specific areas for improvement.
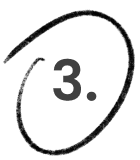
Our analysis suggests that Front aims to enhance efficiency through rule-based automated tagging and predefined templates. Despite these features, users still dedicate significant time to these key tasks:
1.
Crafting responses, while often using ChatGPT for help.
2.
Sorting messages by customer priority and urgency
3.
Assigning messages to the appropriate team members.
Focus on enhancing the automation capabilities to better assist with message assignment and the creation of personalized responses. These enhancements will reduce the time spent on manual tasks, allowing teams to focus more on strategic initiatives and high-level interactions, further enhancing customer satisfaction and efficiency.
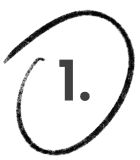
Imagine a spectrum: on one end, we have the current tools where a customer service team receives messages from all channels in one place and AI assists in routing and drafting responses. On the other end, we envision a state of complete automation, where customers are consistently happy and satisfied.
What is every single step in between these two spectrums?
Human Work: Customer service representatives manually handle most queries but use AI for basic tasks like ticket tagging and routing.
AI Work: AI performs simple classification tasks based on predefined rules.
Human Work: Representatives oversee AI-generated responses and routing, making corrections as needed.
AI Work: AI not only routes tickets but also drafts responses based on past interactions and learned patterns.
Human Work: Humans intervene in complex cases or when AI struggles with context.
AI Work: AI manages most interactions, understanding context better and handling a broader range of queries.
Human Work: Human effort shifts towards strategic oversight and handling highly complex or sensitive situations.
AI Work: AI predicts issues before they become problems and initiates proactive customer support interactions.
Human Work: Humans focus on quality control, training AI, and refining AI operations.
AI Work: AI handles all standard customer interactions autonomously, using advanced natural language processing and machine learning.
Human Work: Minimal, focused on system oversight and handling exceptional cases.
AI Work: AI adapts to individual customer preferences and history, personalizing interactions.
Human Work: Almost none, limited to monitoring AI for ethical and functional anomalies.
AI Work: AI handles all aspects of customer service, including complex decision-making, empathy, and conflict resolution.
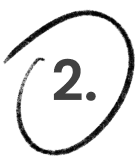
Currently, Front is at Step 1. How can we advance to the next Step?
In addition to the AI Summary, which delivers insights into the conversation, gauges customer satisfaction, and suggests next steps, envision AI automatically assigning messages to the appropriate team member while simultaneously preparing responses ready for review and dispatch.
This streamlined process is feasible with today’s vector-based AI technology. By leveraging a dataset of 50,000 previously handled emails, we can train the AI to understand and categorize email content, converting these into vectors that capture the essential features of each interaction.
We can integrate:
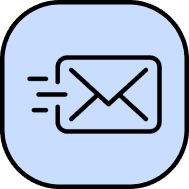
Automated message assignment
Automatically assign incoming messages to the appropriate team member based on previous interactions.
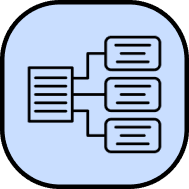
Contextual response generation
Use historical data to generate contextually appropriate responses. The team member only needs to review and send.
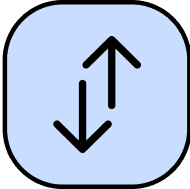
Priority-based message sorting
Organize and sort messages based on the importance of the client, the urgency of the message, and customer satisfaction levels.
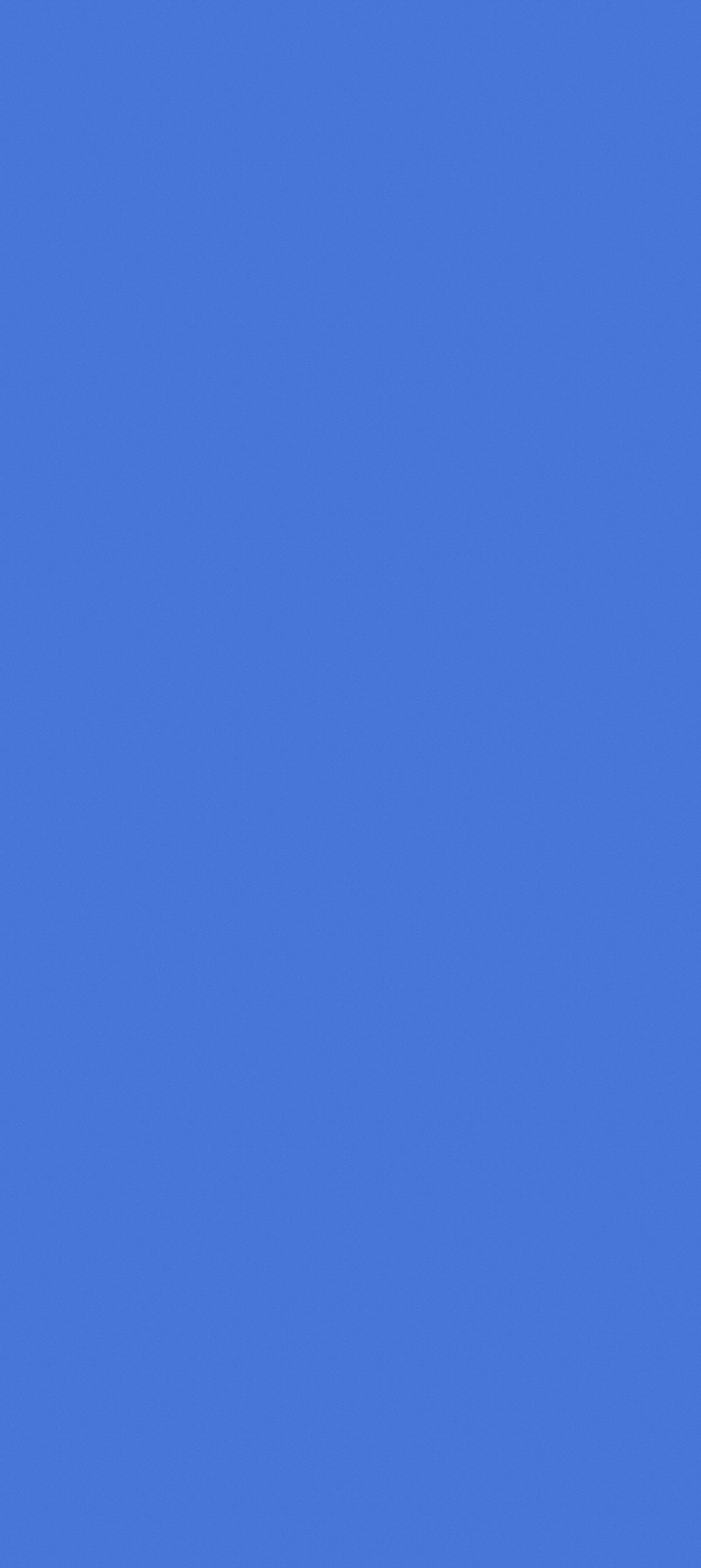
This project required an extensive analysis of the existing customer service communication tools to assess their capabilities and pinpoint areas for improvement. My research led to innovative proposals for integrating advanced AI features that could dramatically enhance the speed and efficiency of the customer support process.
The project was challenging but rewarding, as it allowed me to improve my product design skills and create meaningful enhancements that promise to optimize system performance and enhance user experience.