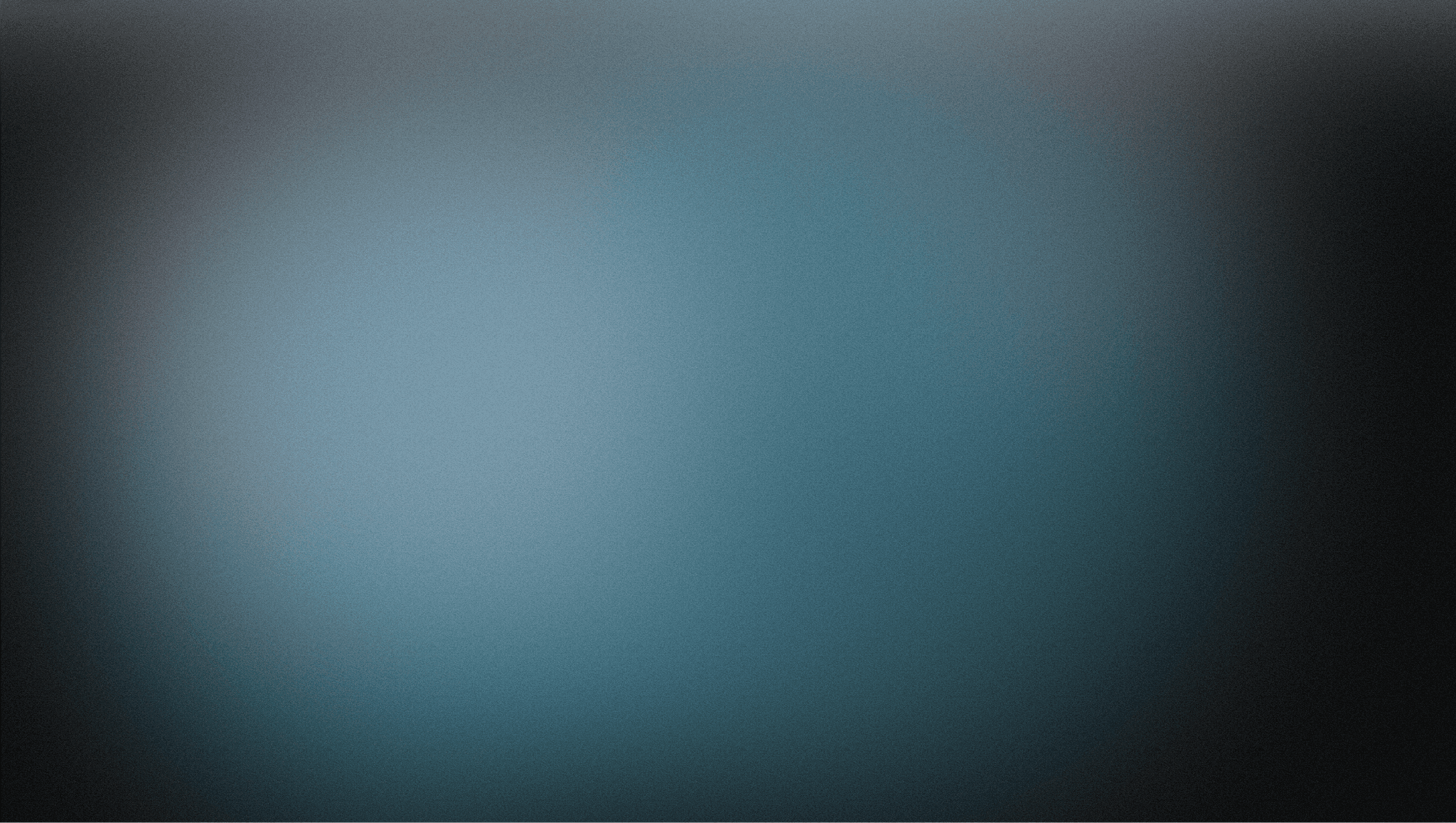
In today's competitive job market, effective compensation planning is crucial for attracting and retaining top talent. Pave, a data-driven compensation platform, aims to revolutionize how companies approach compensation decisions. By providing real-time salary and equity benchmarks, managing compensation bands, and running seamless merit cycles.
However, many companies struggle with losing talent. Research shows that 41% of the global workforce consider leaving their current employers within the next year. Factors such as toxic work environments, lack of professional growth, and underpayment often drive these early job changes.
That is why I decided to help companies retain talent by improving Pave's design.
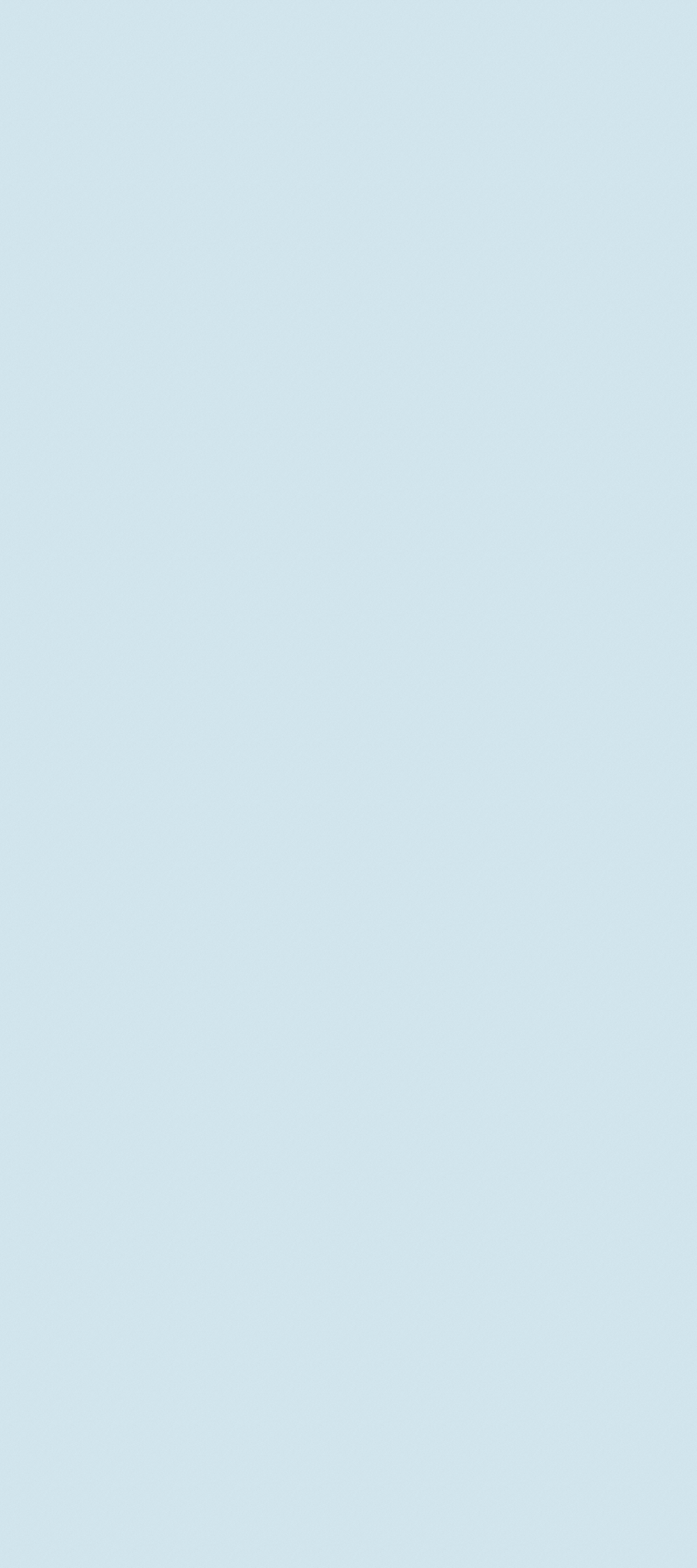
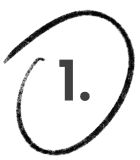
Pave's users include HR professionals, Compensation Managers, Finance Teams, and Executives at fast-growing startups and tech firms. They need to make quick and fair compensation decisions based on budget to retain talent.
The main goal for using compensation tools is to retain talent and plan budgets effectively. These tools help companies achieve several key objectives:
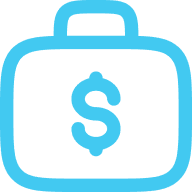
Ensure that the organization offers competitive salaries and benefits to attract and retain top talent.
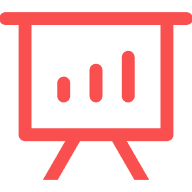
Make informed compensation decisions based on accurate and comprehensive data.
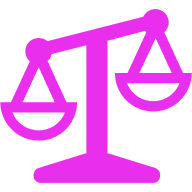
Maintain pay equity across the organization to ensure fairness and compliance with legal standards.
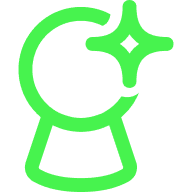
Identify employees at high risk of turnover and anticipate future compensation needs.
1.
Difficulty benchmarking salaries and addressing pay disparities.
2.
Time-consuming and complex compensation data analysis.
3.
Challenges predicting turnover and aligning retention strategies.
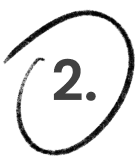
Pave operates in a competitive market with strong players in compensation management. The key challenge is developing better tools to identify and reduce turnover risk while ensuring fair, competitive compensation to retain top talent and stay competitive.
Provides end-to-end compensation software for managing salary ranges, benchmarks, merit cycles, and compliance. This directly overlaps with Pave's offerings in compensation management and planning.
Offers AI-powered compensation software that provides salary data and analytics. Similar to Pave, PayScale focuses on delivering accurate compensation insights and benchmarking.
While primarily an equity management platform, Carta also integrates with compensation planning, making it a direct competitor in the realm of managing total compensation packages.
Provides compensation data and career leveling information for tech roles. Strengths: Offers detailed compensation reports, salary calculators, and real-time percentiles. Provides negotiation support and verified salary data.
Radford provides compensation data and insights for various industries. Strengths: In-depth compensation data and benchmarking for multiple sectors.
Based on the identified challenges and our competitor analysis, these are the specific improvements we can implement:
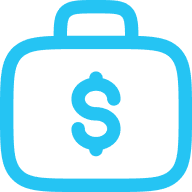
Offer real-time salary band information with employee compensation details for quick, informed adjustments.

Provide tools to analyze compensation data and identify pay gaps, promoting fair pay practices.
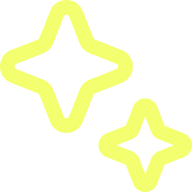
Integrate predictive analytics to identify high-risk employees and enhance analytics tools for better decision-making.
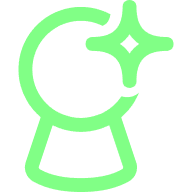
Provide automated recommendations for salary adjustments, promotions, and hiring offers based on benchmark data for efficient decision-making.
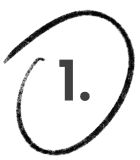
Recent investments in AI-driven HR technologies have produced advanced algorithms, enabling systems to adapt and improve, resulting in predictive analytics and insights.
Why HR professionals are one of the main users of AI:
Improved efficiency and productivity
Reduced costs
Fewer repetitive and time-consuming tasks
More data-driven decision-making
of global 2000 companies are expected to use algorithmic managers for hiring, firing, and training workers.
*IDC’s Future of Work research
of HR departments are currently using AI for payroll processing and benefits administration.
*IDC’s Future of Work research
of HR departments are using AI for managing employee records.
*The Future of Work survey by Eightfold AI
As companies adopt AI, leveraging these advancements is essential to stay competitive. AI enhances our ability to achieve compensation goals with advanced tools and insights.
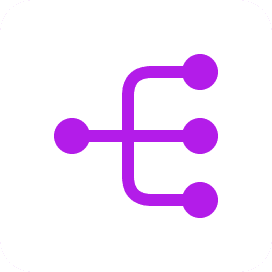
AI analyzes extensive data, including market benchmarks, employee information, and performance metrics, comparing factors like gender, location, and position.
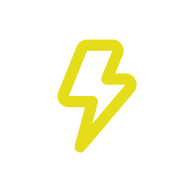
AI handles pattern identification, budget calculations, and high-risk employee assessments efficiently, providing clear, budget-conscious recommendations.
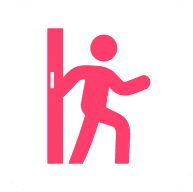
AI predicts employee turnover risk by assessing position popularity in specific locations and analyzing company employment history for patterns.

AI offers compensation recommendations based on the budget, covering cash, equity, merit budgets, and bonuses.
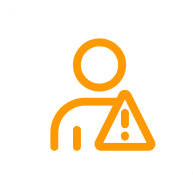
AI identifies high-risk employees and provides summaries of its recommendations, enabling immediate action to retain key talent.
While AI provides advanced tools and insights for compensation analysis, HR professionals need additional key metrics to finalize their decisions:
By integrating this additional information with AI analysis, HR professionals can make well-rounded, data-driven compensation decisions that are fair, competitive, and aligned with organizational goals.
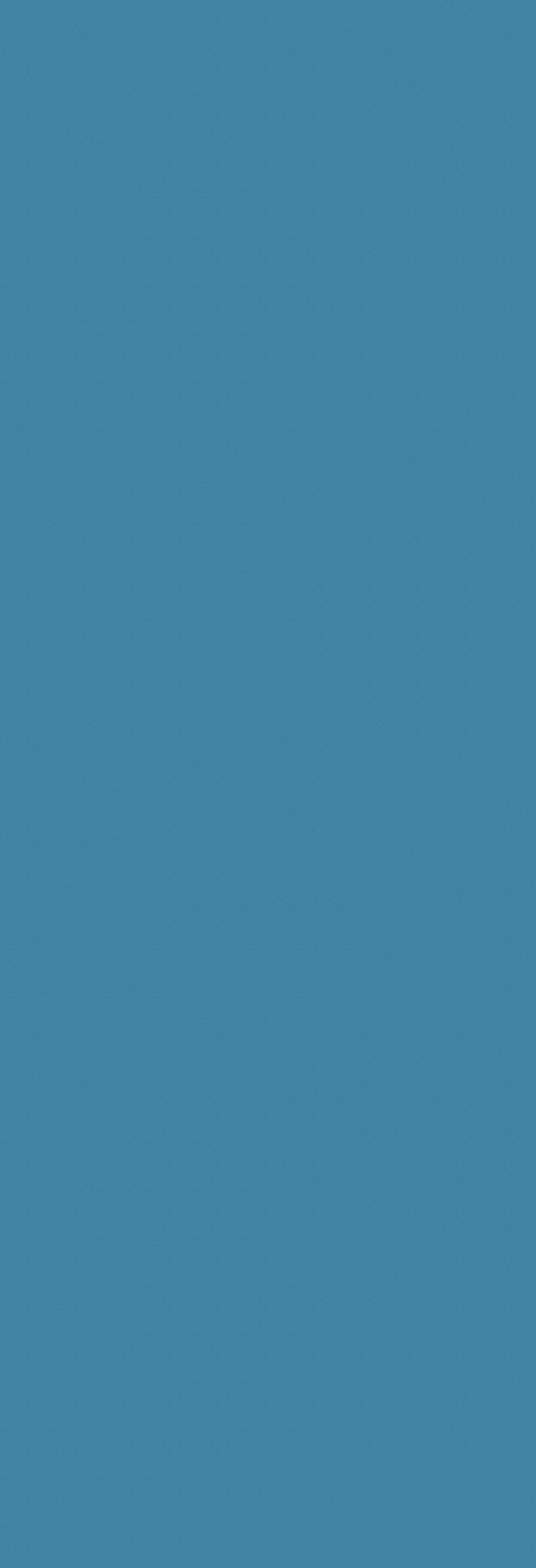
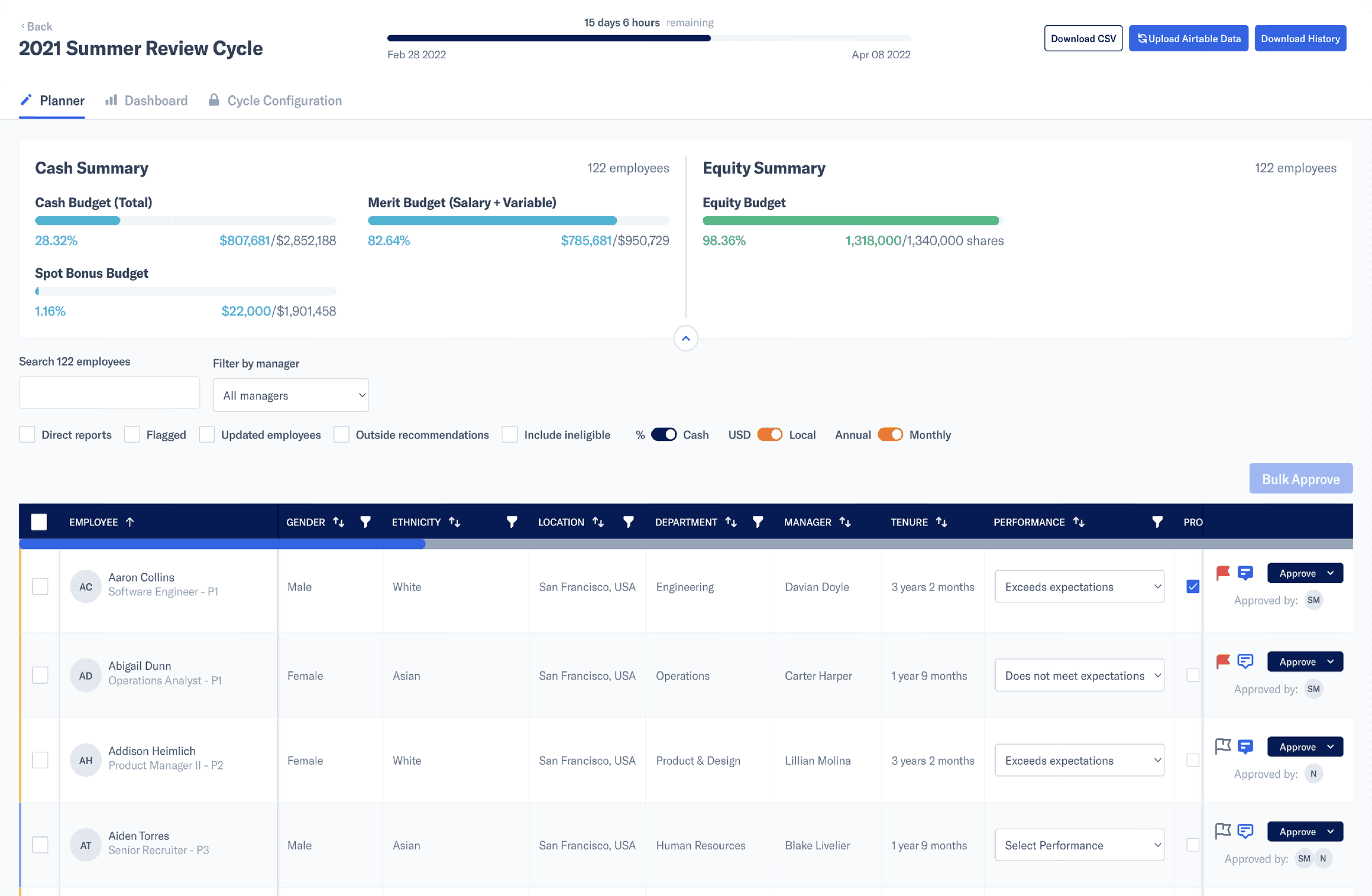
In this case study, I explored how AI can improve Pave's compensation planning. By addressing key challenges, I aimed to make decision-making more efficient for HR and finance teams. Integrating real-time salary data, predictive analytics, and automated recommendations helps ensure fair pay and retain top talent.
This personal project shows how AI can transform compensation management, making Pave's tools more dynamic and competitive. By implementing these ideas, Pave can better serve its users and stay ahead in the job market.